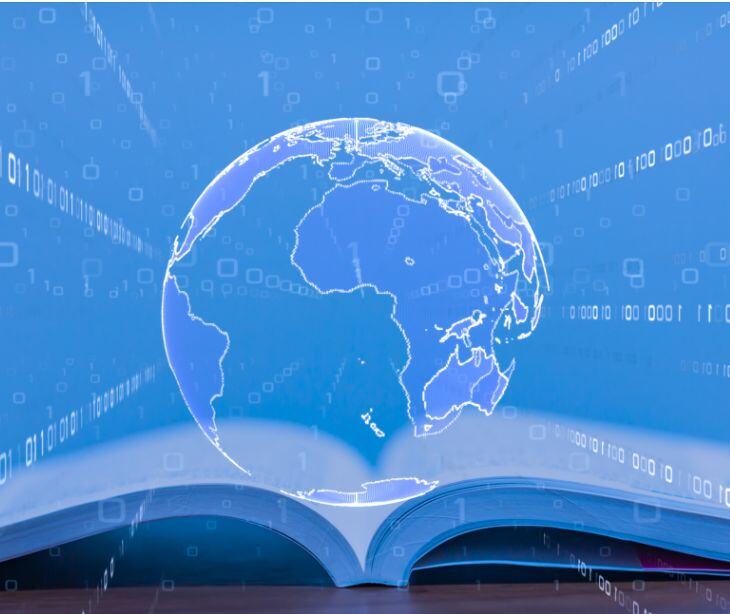
Large language models (LLMs) are AI systems that analyze unstructured text—such as doctor’s notes, lab reports, and medical literature—to help healthcare providers make faster and more informed decisions. However, integrating LLMs into clinical workflows raises questions about data privacy, ethical use, and practical implementation.
Understanding the evolution of medical LLMs
Large language models have come a long way since their early days as simple chatbots. Their transformation can be traced through notable milestones in natural language processing (NLP) technology, which has shaped their growing role in healthcare:
- 1960s: Early NLP systems focused on translating medical terms into codes for databases.
- 1980s: Expert systems using rule-based NLP began assisting doctors with decision-making.
- 2000s: Statistical methods in NLP improved the interpretation of electronic health records (EHRs).
- 2013: The introduction of Word2Vec allowed machines to understand the context and meaning of text data.
- Late 2010s: Transformer-based models, such as BERT (Bidirectional Encoder Representations from Transformers), and GPT, revolutionized NLP by improving context understanding.
The shift from pre-trained language models (PLMs) like BERT to sophisticated LLMs like GPT-3 and GPT-4 has expanded the potential applications of AI in healthcare. These newer models excel at processing vast amounts of unstructured data, including medical notes, lab reports, and scientific literature, making them valuable tools for healthcare professionals.
Large language models in healthcare: Core capabilities
Processing unstructured data
LLMs stand out from traditional machine learning models by analyzing unstructured medical text, such as physician notes, patient histories, and clinical trial reports. Their ability to derive insights from unstructured data expands the potential for innovation in healthcare applications.
Understanding medical language
Unlike general-purpose LLMs, medical-specific models are trained on large-scale biomedical corpora. These specialized models understand the nuances of medical terminology and can generate more accurate responses in clinical contexts.
Generating human-like responses
LLMs can engage in natural conversations with patients and healthcare providers, making them useful for chatbots, virtual assistants, and patient education tools.
Read also:
Diverse applications of LLMs in healthcare
Medical large language models are used across various healthcare domains to improve efficiency, accuracy, and patient outcomes. Here are some applications:
Clinical decision support
LLMs can assist healthcare providers in diagnosing diseases, recommending treatments, and predicting patient outcomes. For example:
- Glass Health: This clinical LLM suggests possible diagnoses and treatment plans based on patient summaries, providing invaluable support in clinical decision-making.
- NYU Langone Health: In collaboration with NVIDIA, NYU developed NYUTron, an LLM that predicts patient readmissions within 30 days of discharge.
Automating routine tasks
LLMs can automate time-consuming administrative tasks, freeing up healthcare professionals to focus on patient care. For example:
- Automating appointment scheduling and medical data entry
- Summarizing medical notes into structured EHR fields
- Transcribing provider-patient consultations into detailed SOAP notes
Personalized patient communication
LLMs enhance patient engagement by providing personalized responses based on a patient’s medical history, symptoms, and concerns. This is particularly useful in:
- Remote patient monitoring (RPM) chatbots
- Mental health support chatbots that offer empathetic, real-time assistance
- Patient education tools that explain complex medical concepts in simple terms
Medical research
LLMs can accelerate medical research by analyzing large datasets, identifying trends, and generating hypotheses. For instance:
- Using LLMs to identify potential treatments for rare diseases
- Optimizing clinical trial design to reduce costs and improve success rates
Drug discovery and clinical trials
LLMs can analyze scientific literature and predict drug interactions, helping researchers identify new drug targets and optimize trial design. In early 2020, Exscientia became the first company to bring an AI-designed drug molecule into human clinical trials, demonstrating the potential of LLMs in drug discovery.
While large language models are already transforming certain aspects of healthcare, recent research reveals notable gaps in how these tools are being evaluated. A systematic review published in JAMA analyzed over 500 studies on LLMs in healthcare, showing that most evaluations are limited to knowledge-based tasks, such as answering medical exam questions, rather than patient care or administrative workflows. Surprisingly, only 5% of the studies involved real patient data, indicating a gap between theoretical testing and real-world applications.
The review also noted that while LLMs are being tested for clinical decision-making and text classification, tasks like conversational dialogue, summarization, and administrative support, areas with the potential to reduce provider burden remain underexplored. Additionally, evaluations often focus on accuracy (over 95%) but pay little attention to ethical issues like bias or toxicity. This inconsistency indicates the need for a more structured approach to testing LLMs in healthcare, particularly across different specialties and administrative processes, to ensure these tools can safely and effectively improve patient care and operational efficiency.
Real-World Case Studies of Medical LLMs
Several healthcare organizations have successfully integrated large language models into their workflows, yielding impressive results:
Epic and Microsoft
Epic, a leading EHR provider, partnered with Microsoft to integrate GPT-4 into its system, enabling healthcare providers to identify trends in medical data and personalize patient care through advanced AI capabilities.
Google’s AIME (AI for Medical Empathy)
Google’s conversational AI model, AIME, is designed to facilitate empathetic conversations with patients and assist in diagnostic questioning. A recent study found that AIME outperformed human benchmarks in 28 of 32 assessment criteria.
Beth Israel Deaconess Medical Center
Researchers at Beth Israel discovered that ChatGPT-4 improved diagnostic performance, particularly when lab test results were included. This proves the model’s potential to enhance clinical decision-making.
Challenges with implementing medical LLMs
Despite their potential, implementing LLMs in healthcare comes with challenges:
- Data privacy and security: LLMs must comply with data protection regulations like HIPAA to ensure patient privacy. Techniques such as encryption, federated learning, and data de-identification can help address privacy concerns.
- Model transparency: Understanding how an LLM makes decisions can be challenging. The lack of transparency can affect trust and accountability in clinical settings.
- Bias and fairness: LLMs may inherit biases from their training data, leading to disparities in healthcare delivery. Ensuring diverse and representative training data is necessary to mitigate bias.
- Integration with existing systems: Integrating LLMs into complex healthcare systems can be difficult. Ensuring seamless integration without disrupting workflows is beneficial for their success.
Ethical and legal considerations for medical LLMs
Healthcare organizations must tackle ethical and legal challenges when implementing LLMs:
- Complying with HIPAA: LLMs must protect patient data by ensuring secure data storage and transmission.
- Addressing ethical concerns: Organizations should follow ethical guidelines, such as those outlined by the World Health Organization (WHO), to ensure transparency, accountability, and inclusivity.
FAQs
Which large language models are commonly used in healthcare?
Examples of large language models utilized in healthcare include BioBERT, ClinicalBERT, GPT-3, GPT-4, GatorTron, Med-PaLM2, HuatuoGPT, XLNet, and ClinicalGPT. These models have demonstrated potential across various healthcare applications.
How do large language models enhance patient care?
Large language models can enhance patient care by supporting clinical decision-making, streamlining administrative processes, analyzing medical records to detect patterns, enabling personalized patient interactions, and contributing to medical research and clinical trials.
Subscribe to Paubox Weekly
Every Friday we'll bring you the most important news from Paubox. Our aim is to make you smarter, faster.