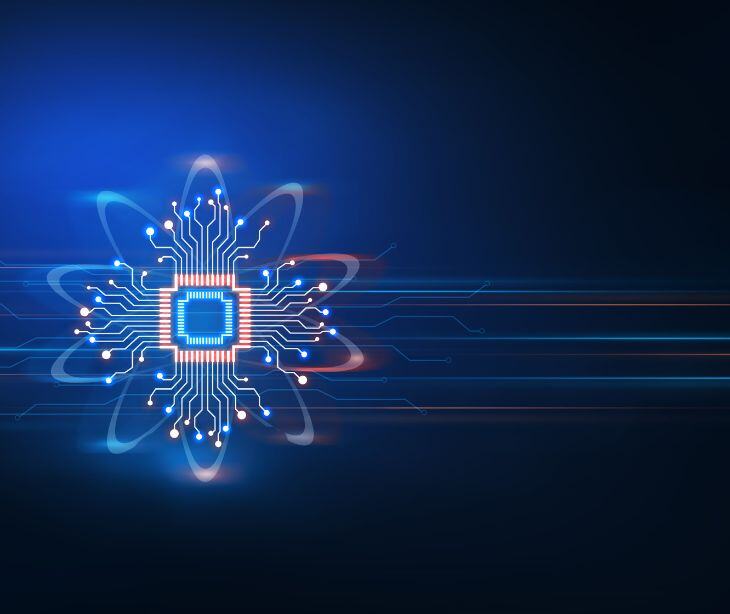
The healthcare industry is undergoing a profound transformation, driven by the rapid advancements in technology and the exponential growth of data. At the forefront of this revolution is the integration of machine learning, a powerful subset of artificial intelligence poised to redefine modern medicine's landscape. As patient data becomes increasingly accessible and complex, healthcare professionals are recognizing the immense potential of machine learning to extract meaningful insights, enhance diagnostic accuracy, personalize treatment plans, and ultimately improve patient outcomes.
Understanding machine learning in healthcare
Machine learning is a technological marvel that enables systems to learn and adapt without explicit programming. Unlike traditional software, which relies on predefined rules and algorithms, machine learning algorithms can analyze data, identify patterns, and make autonomous decisions. In the healthcare domain, this capability is particularly valuable, as it allows for the processing and interpretation of the massive volumes of medical data generated daily within electronic health records.
Read more: What is machine learning?
Transforming diagnostic accuracy with machine learning
One of the most promising applications of machine learning in healthcare is its ability to enhance diagnostic accuracy. Machine learning algorithms can be trained to analyze medical images, such as X-rays, MRI scans, and pathology slides, with remarkable precision. These algorithms can detect patterns and identify subtle anomalies that may be challenging for human experts to discern, leading to earlier and more accurate diagnoses.
Automated image analysis
Machine learning models, particularly deep learning algorithms, have demonstrated exceptional performance in interpreting medical images. These models can rapidly analyze vast datasets of images, identifying features and patterns that may indicate the presence of specific diseases or conditions. This automated image analysis can assist healthcare providers in making more informed and timely decisions, ultimately leading to improved patient outcomes.
Personalized diagnosis and treatment
Beyond image analysis, machine learning can also be used to personalize diagnosis and treatment plans. By analyzing an individual's medical history, genetic data, and other relevant factors, machine learning algorithms can identify unique patterns and risk factors, enabling healthcare providers to develop customized treatment strategies that cater to the specific needs of each patient.
Related: Personalized patient education, HIPAA, and AI
Improving healthcare operational efficiency
In addition to enhancing clinical outcomes, machine learning in healthcare can also drive improvements in operational efficiency, leading to cost savings and more streamlined patient-centric processes:
Automating administrative tasks
Machine learning can be used to automate a wide range of administrative tasks, such as medical billing, appointment scheduling, and patient record management. By automating these repetitive and time-consuming processes, healthcare organizations can free up valuable resources, allowing healthcare professionals to focus on providing high-quality patient care.
Predictive analytics for resource optimization
Machine learning algorithms can also be used to analyze historical data and predict future trends, enabling healthcare organizations to optimize the allocation of resources, such as staffing, equipment, and supplies. This predictive analytics approach can help healthcare providers anticipate and respond to fluctuations in demand, ensuring that patients receive the care they need when they need it.
Enhancing patient engagement and outcomes
Beyond the operational and clinical benefits, machine learning in healthcare can also enhance patient engagement and improve overall patient outcomes:
Personalized patient monitoring
Machine learning algorithms can be integrated with wearable devices and remote monitoring systems to continuously track patient data, such as signs, activity levels, and medication adherence. By analyzing this data, healthcare providers can proactively identify potential health issues and intervene before they escalate, leading to better patient outcomes and reduced hospital readmissions.
Predictive analytics for patient risk assessment
Machine learning models can also analyze patient data, including electronic health records, to predict the risk of developing specific health conditions or complications. This risk assessment capability can enable healthcare providers to implement targeted preventive measures and personalized treatment plans, ultimately improving patient outcomes and reducing healthcare costs.
Read also: Defining personalized care
Addressing Ethical Considerations and Data Privacy Concerns
As machine learning continues to transform the healthcare industry, it is necessary to address the ethical and data privacy considerations that come with the increased use of this technology:
Ensuring transparency and accountability
Healthcare organizations must prioritize transparency and accountability when implementing machine learning systems. This includes clearly communicating the purpose and limitations of these technologies, as well as establishing governance frameworks to ensure the responsible and ethical use of patient data.
Protecting patient privacy and data security
The integration of machine learning in healthcare also heightens the imperativeness of data security measures. Healthcare providers must implement stringent data protection protocols, such as encryption, access controls, and regular security audits, to safeguard patient information and maintain public trust.
Read more: What is healthcare data security?
In the news
The WHO's recent report discusses regulating AI in healthcare. It brings to attention the broader challenges and potential risks associated with AI in various sectors, including ethical concerns, data privacy, and the amplification of biases. As the WHO identifies main focus areas like transparency, risk management, data quality, and privacy protection in healthcare AI, it brings to attention the need for oversight in all AI applications. The involved companies are required to respond within 45 days of receiving the order, marking a major moment in the regulation and oversight of the AI sector.
See more: WHO releases publication outlining considerations for AI in healthcare
FAQs
How is machine learning used in healthcare?
ML is used to analyze complex medical data, predict patient outcomes, personalize treatment plans, automate administrative tasks (such as scheduling and billing), and assist in medical imaging interpretation.
What are the benefits of machine learning in healthcare?
ML improves diagnostic accuracy, supports early disease detection, enhances treatment planning through personalized medicine approaches, optimizes resource allocation, and facilitates clinical decision-making based on large datasets.
What are the challenges of implementing machine learning in healthcare?
Challenges include ensuring data quality and interoperability across healthcare systems, addressing issues of bias and interpretability in ML models, integrating with existing clinical workflows, managing regulatory compliance, and securing patient data.
Learn more: HIPAA Compliant Email: The Definitive Guide
Subscribe to Paubox Weekly
Every Friday we'll bring you the most important news from Paubox. Our aim is to make you smarter, faster.