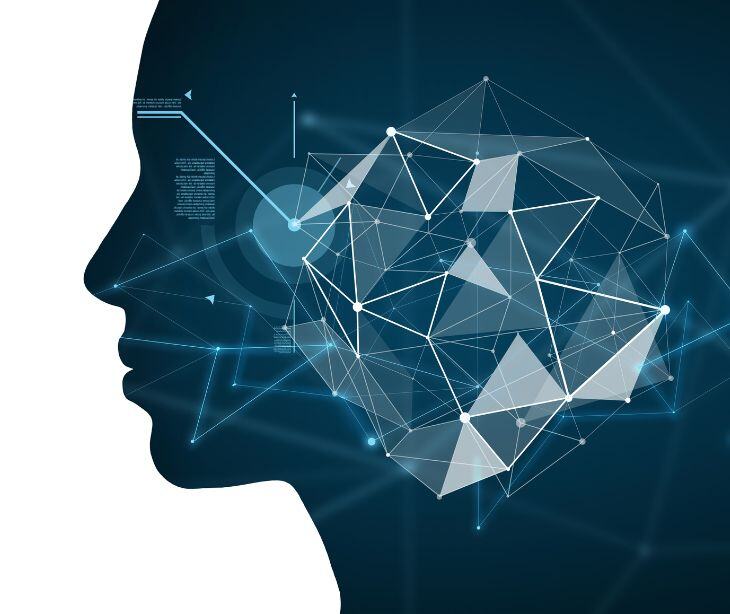
“Global cyber attacks continue to rise in 2024, with the average number of cyber attacks per organization per week reaching 1,308 in the first quarter of 2024. This is a 28% increase from the last quarter of 2023 and a 5% increase year-over-year,” says Secureframe. The increase in cyberattacks leads to an increase in the costs of these attacks. In 2023, cybercrime losses reached a new peak of $12.8 billion and are projected to skyrocket to $23.84 trillion by 2027.
These statistics are alarming and emphasize the importance of cybersecurity.
One of the most significant developments in recent years has been the integration of artificial intelligence (AI) into cybersecurity strategies. AI, with its ability to analyze vast amounts of data and identify patterns, offers unprecedented capabilities for both defense and offense in the realm of digital security.
The intersection between AI and cybersecurity
Artificial intelligence (AI) is significantly impacting cybersecurity in various ways, enhancing both defense mechanisms and the sophistication of cyber threats. Here are some key areas where AI is making a difference:
Threat detection and prevention
- Anomaly detection: AI algorithms analyze network traffic and user behavior to identify anomalies that might indicate potential security threats, such as malware, phishing attempts, or unauthorized access.
- Intrusion detection systems (IDS): AI-powered IDS can automatically detect and respond to suspicious activities, often with greater accuracy and speed than traditional systems.
- Malware detection: Machine learning models can analyze patterns in software behavior to detect new and evolving malware, including zero-day threats.
Automated response and incident management
- Automated threat response: AI systems can automatically respond to certain types of cyber threats, such as isolating infected systems, blocking malicious IP addresses, or applying patches, thereby reducing response times.
- Incident analysis: AI helps in the triage process by quickly analyzing vast amounts of data to identify the root cause of an incident and recommend remediation steps.
Predictive analytics and threat intelligence
- Threat prediction: By analyzing historical data and identifying trends, AI can predict potential future attacks and vulnerabilities, allowing organizations to strengthen their defenses proactively.
- Threat intelligence gathering: AI automates the collection and analysis of threat intelligence from various sources, providing real-time updates on emerging threats and attack vectors.
Fraud detection and prevention
- Behavioral analysis: AI systems monitor user behavior to detect deviations from the norm, which can indicate fraudulent activities such as account takeovers or unauthorized transactions.
- Real-time monitoring: AI enables real-time monitoring and detection of fraudulent activities across various platforms, including financial services, e-commerce, and online transactions.
Worth reading: Using behavioral analytics in HIPAA compliant email marketing
Security automation and orchestration
- Security orchestration: AI integrates and automates various security tools and processes, improving the efficiency and effectiveness of security operations centers.
- Automation of routine tasks: AI can take over repetitive and mundane security tasks, freeing human analysts to focus on more complex and strategic issues.
User and entity behavior analytics (UEBA)
- Enhanced monitoring: AI-based UEBA systems analyze the behavior of users and entities within the network to detect insider threats and compromised accounts.
- Risk scoring: AI assigns risk scores to users and devices based on their behavior, helping prioritize security efforts and resource allocation.
Adversarial AI and countermeasures
- AI-driven attacks: Cybercriminals are also leveraging AI to enhance the sophistication of their attacks, such as by creating highly convincing phishing emails or evading traditional security measures.
- Counter-adversarial AI: Security teams use AI to develop countermeasures against AI-driven attacks, ensuring that defenses evolve in tandem with the threats.
Natural language processing (NLP)
- Threat analysis: NLP helps in the analysis of text-based data, such as threat reports, social media feeds, and communication logs, to identify and understand potential threats.
- Automated alerts: AI can parse and understand human language to generate automated alerts and summaries for security analysts, improving their ability to respond swiftly.
Challenges and considerations
- False positives/negatives: AI systems can sometimes produce false positives or false negatives, requiring continuous tuning and validation.
- Data privacy: The use of AI in cybersecurity involves the processing of large amounts of sensitive data, raising concerns about data privacy and compliance with regulations.
- Adversarial attacks: AI systems themselves can be targeted by adversarial attacks designed to mislead or corrupt their functioning.
See also:
FAQs
What is artificial intelligence (AI)?
AI refers to the simulation of human intelligence in machines that are programmed to think and learn like humans. These systems can perform tasks that typically require human intelligence, such as visual perception, speech recognition, decision-making, and language translation.
What are the challenges in implementing AI?
Challenges in implementing AI include data quality and availability, computational resources, technical expertise, ethical considerations, and integration with existing systems.
Subscribe to Paubox Weekly
Every Friday we'll bring you the most important news from Paubox. Our aim is to make you smarter, faster.